Students 2022
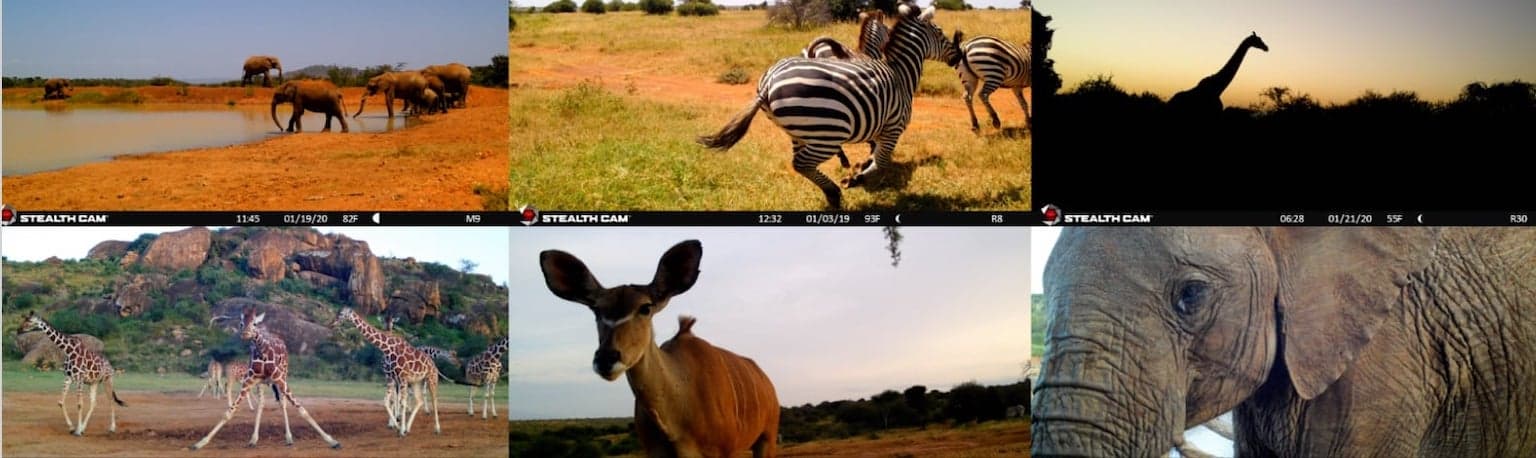
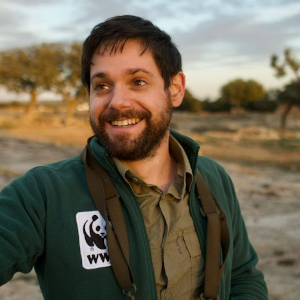
Antón Álvarez (@antonalvarezbc)
WWF SpainI am Antón Álvarez, a conservation biologist, student_currently the technical coordinator of Iberian lynx projects at WWF Spain. Within conservation biology, I have always tried to be pragmatic. This has led me to work on several endangered species, such as the Iberian lynx, the giant otter and the pink river dolphin, among others, and always with a clear objective: to try to apply statistical and technological development to the study and conservation of the biodiversity. I am aware that interdisciplinary collaborations are essential to reach solutions that have a real impact. I believe that thanks to CV4ecology, I will be able to collaborate much more in the near future.
Project: The Iberian lynx is the most endangered feline and one of the least genetically diverse species. Therefore, it is crucial to identify when a lynx ID from one subpopulation moves to another, enabling gene flow between subpopulations. Thanks to conservation efforts, the lynx population has grown from around 100 individuals in 2002 to 1365, and its distribution has increased in the last few years. As a result, the re-ID work (individual identification of each lynx from a picture) has grown exponentially. My project will focus on updating the re-ID algorithms to state-of-the-art. Ideally, this development will impact Iberian lynx conservation, as they can be implemented in platforms such as Wildbook and used to contribute to censuses or detect movements between subpopulations.
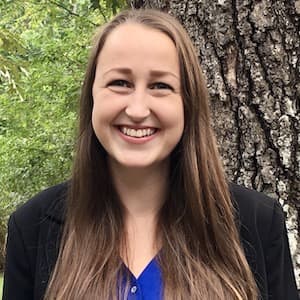
Carly Batist (@Carly_Batist)
City University of New York (CUNY)I am a PhD Candidate in Biological Anthropology/Primatology at the CUNY Graduate Center (born-and-raised New Yorker as well!). I study black-and-white ruffed lemur vocalizations and how passive acoustic monitoring and machine learning can be used in conservation efforts for this Critically Endangered species. I conduct this research in the southeastern Madagascar rainforests in collaboration with Centre ValBio research station. I also co-maintain the Conservation Tech Directory website. I have a B.S. in Animal Science from Cornell University and a M.S. in Primate Behavior from Central Washington University. When I'm not wearing my PhD hat, I love to horseback ride, hike and will the NY Giants & Arsenal to win.
Project: I use passive acoustic monitoring (PAM) to survey ruffed lemur populations in southeastern Madagascar. I’m deploying PAM recorders (Audiomoths) at sites across a 120km rainforest corridor where little to no research has taken place since the 1980s and for which we don’t know the distribution lemurs. For the CV4E workshop, I’ll be using machine learning to automatically detect/pick out ruffed lemur calls in spectrograms (2-D image representations of sound) from my long-term PAM recordings. These detections, combined with the spatial layout (GPS) of the devices, can then be used to model lemur distribution and range in the corridor. This will contribute to the next species census and help identify sites to prioritize for future conservation initiatives.
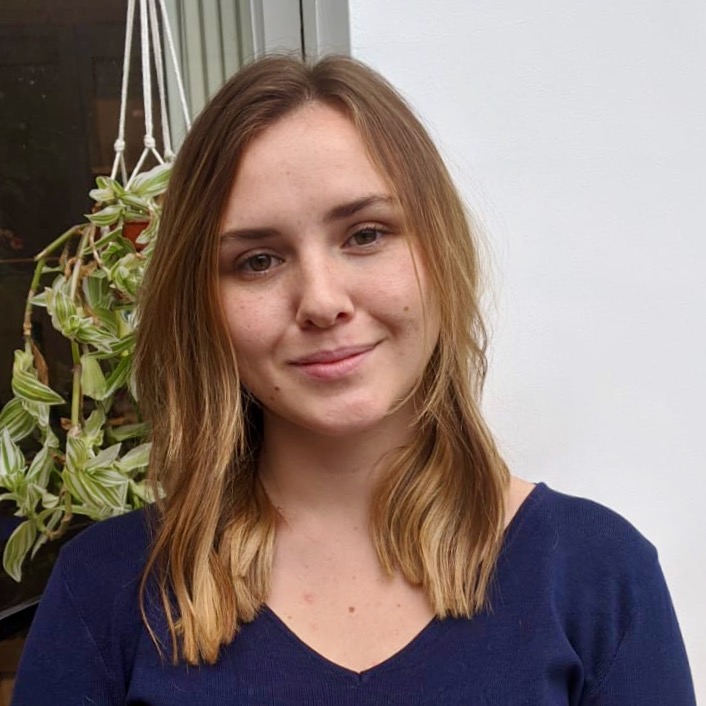
Peggy Bevan (@PeggyBevan2)
University College LondonI'm Peggy, a third year PhD student from University College London. I have a degree in Zoology and went on to complete a Research Masters in Biodiversity, Conservation and Evolution at UCL. Our ecosystems and biodiversity are integral to human existence, and my interest in biodiversity monitoring comes from a passion to keep healthy and thriving environments. My PhD uses novel technologies like camera traps and acoustics to consider how best to monitor biodiversity. I use data from a field site in the terai region of Nepal to look at how human disturbance is impacting wildlife there, and am developing best practices for ecosystem monitoring.
Project: During the CV4E summer workshop, I plan to ask if we can infer ecosystem health from camera trap images, without identifying species. I plan to use cutting-edge unsupervised machine learning techniques to look for features in images that might correlate with species richness, diversity or other indicators of ecosystem health.
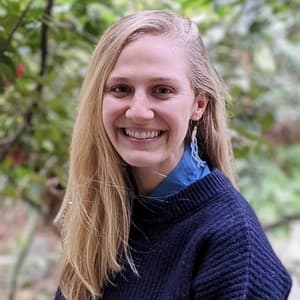
Catherine Breen (@katiembreen)
University of WashingtonCatherine Breen is a PhD student and NASA Fellow at the University of Washington. She is on the pursuit to use computer science to advance ecology and the wellbeing of wildlife in a changing climate. Her work is supported by NASA, the American Scandinavian Foundation, and Microsoft’s AI for Earth Program. Before coming to UW, Catherine was a remote sensing analyst at NASA Ames Research Center, and the first Energy and Climate Fellow at The Wilderness Society. Believing the best way to understand nature’s complexities is through experience, she thru-hiked the full Pacific Crest Trail in 2019. Catherine Breen has an Ecology and Evolutionary Biology degree from Princeton University.
Project: Changing snow conditions are likely to have a major impact on northern wildlife, but climate change research has focused far more on changing temperatures than precipitation and snow. Warm winters have increased the frequency of midwinter rain events, when rain falls on snow, and these events can create an icy layer, restricting vegetation access for grazers, or melt the snow completely, reducing energetic costs for winter movement. Using a 1000+ camera trap network in Norway, this project will develop a computer vision model that distinguishes rain from snow, and, by incorporating a model that detects snow cover, when it is raining on snow. Distinguishing between rain and snow in wildlife images will accelerate our ability to track rain, snow, rain on snow trends, and subsequent wildlife and biodiversity impacts.
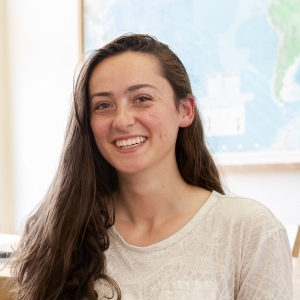
Anna Boser (@BoserAnna)
University of California, Santa BarbaraI am a PhD Student at UCSB's Bren School for Environmental Science and Management, where I study water scarcity in agricultural settings. In a field dominated by mechanistic and physics-based models, I am interested in harnessing data-driven approaches such as satellite remote sensing and machine learning to learn about water use and management in agricultural systems. I graduated from UC Berkeley in 2020 with a BA in statistics, and am excited to bring my data skills to the next level at the CV4Ecology summer school.
Project: One important satellite-based product used for hydrological research is remotely sensed land surface temperature (LST), because this is the most important input for estimating evapotranspiration. However, the coarse resolution of such data has been a persistent problem. I propose leveraging a deep learning approach to generate sharpened satellite-based LST images. While extensive LST super-resolution research has been done at the pixel level, I believe the ability of a convolutional neural network to recognize spatial patterns in the data will allow for an improved product.
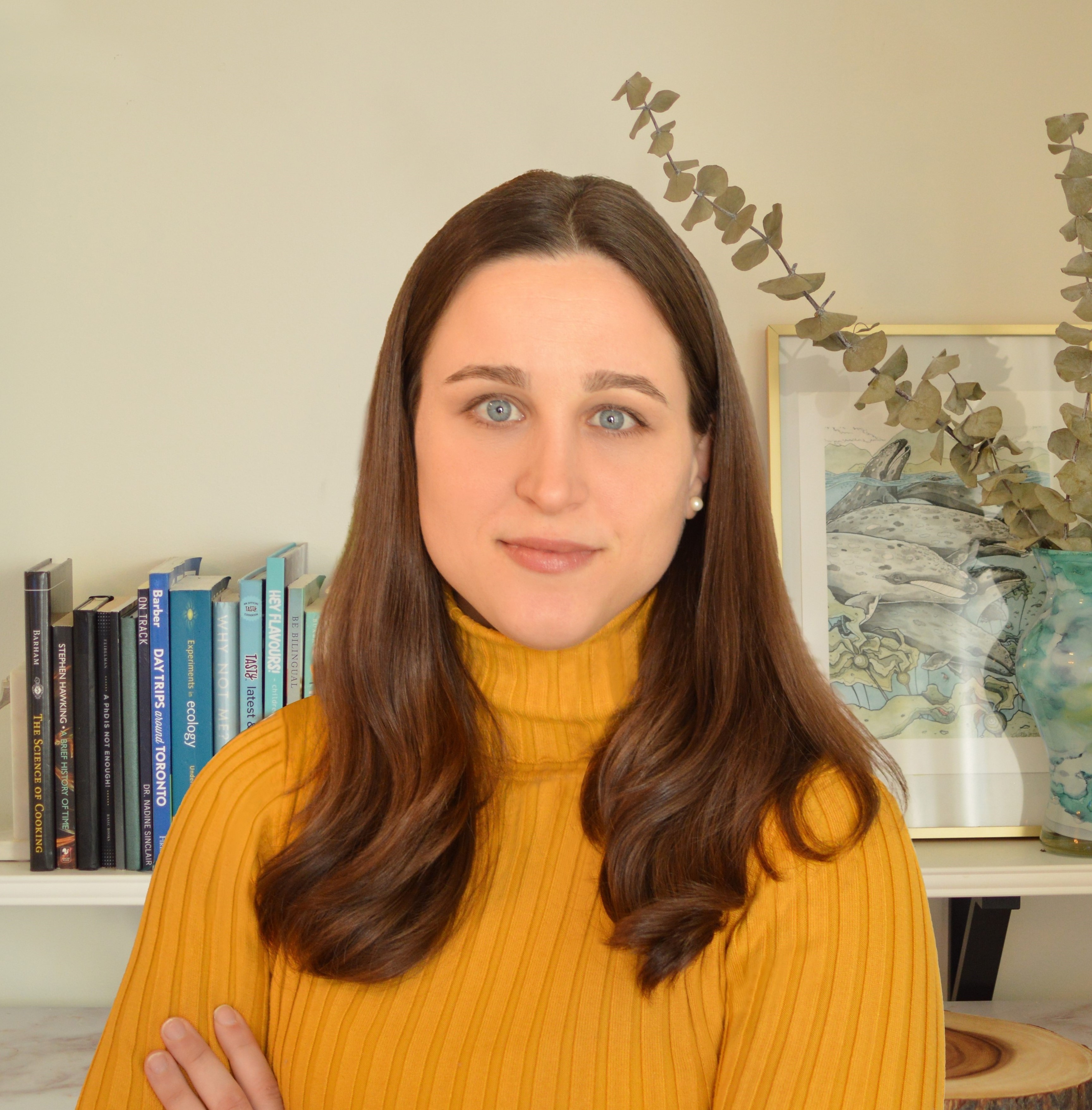
Tiziana Gelmi Candusso (@UrbanZoochory)
University of TorontoTiziana is a postdoctoral research fellow at the University of Toronto working on the spatial ecology of urban wildlife. Using tracking data and camera traps she develops models to understand the presence of carnivores within the city of Toronto, and how their movement patterns potentially influence the health of urban ecosystems through inter-specific interactions. As part of her current research project, Tiziana collects data with camera traps on all urban vertebrate wildlife present in Toronto and collaborates on multi-city analyses across continents through the Urban wildlife information network. Before coming to the University of Toronto she studied animal behavior and its influence on plant gene flow in Germany and currently holds a research fellowship awarded by the German research foundation (DFG).
Project: As a human-dense environment, collecting camera trap data on urban wildlife involves the collection of photos of people, domestic animals, vehicles, and other noise-creating objects, intensifying the work behind managing, storing, and tagging the data. The goal of the project is to train a machine-learning model specific for urban wildlife in temperate ecosystems. In collaboration with the urban wildlife information network, using data collected across the continent and within Toronto, Tiziana aims to create an open-source code that will accelerate the processing of urban wildlife images. An urban-specific and accessible model will facilitate the widespread and long-term collection of presence and absence data of animals in cities, fundamental to assess and address perturbations across urban mammal communities.
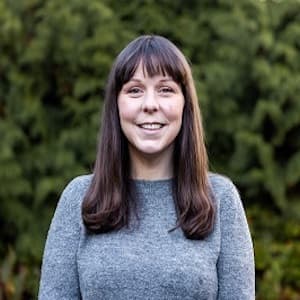
Melanie Clapham (@MelanieClapham)
University of Victoria/BearID ProjectI am a Postdoctoral Research Fellow at the University of Victoria, Canada, within the Applied Conservation Science Lab. I am also a co-founder of the BearID Project, a non-profit research organisation that develops non-invasive technologies to research and monitor bears and other wildlife. My academic interests focus on the social behaviour of bears, particularly research into olfactory communication. I am especially interested in the application of conservation technology to advance field techniques for large carnivore research. Through my applied work, I collaborate with First Nations to aid the inclusion of Western science in wildlife management decisions. I also work closely with ecotourism operators in British Columbia to assess and reduce impacts of commercial viewing on wildlife.
Project: I am interested in automated methods of individual ID for species that lack unique markings. Bears are difficult to research and monitor as they are elusive, inhabit a wide variety of habitats, have large home-ranges, and generally don’t possess stable markings that can be used to identify individuals. Collectively, this restricts the tools and techniques available to study and conserve them. Manual individual ID of unmarked species requires species-specific expertise, population-specific training, and is an extremely time-consuming process. My project will use four years of camera trap data (20 second, 1080p videos) from coastal British Columbia to develop a classification model for individual ID of brown (grizzly) bears using a facial recognition approach. This model would represent the first automated tool for individual ID developed using camera trap imagery for any bear species.
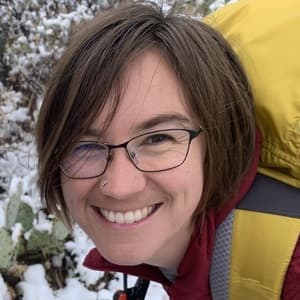
Rowan Converse
University of New MexicoRowan is a PhD student in Geography and Environmental Studies at the University of New Mexico. After spending the early part of her career working as a wildlife technician on endangered species monitoring and recovery programs in Arizona and New Mexico, she pivoted into GIScience to learn how to leverage emerging geospatial tools for wildlife conservation and management. She also supports a variety of imaging and LiDAR collection projects as the UAS pilot for the ASPIRE Center at UNM. In her spare time, she enjoys exploring the beautiful landscapes of the American Southwest through running, cycling, paddleboarding, and cross-country skiing.
Project: Rowan is working with the US Fish and Wildlife Service as the project manager for “Drones for Ducks”, a collaborative project with the aim of automating the annual migratory waterfowl census at federal wildlife refuges in the Southwest Region. The program is developing a framework for surveying waterfowl with UAS and deriving automated population counts through machine learning. The project has been certified on the citizen science platform Zooniverse and has collected over 200,000 image labels since May 2021; to participate or learn more, visit the project site: Drones for Ducks.
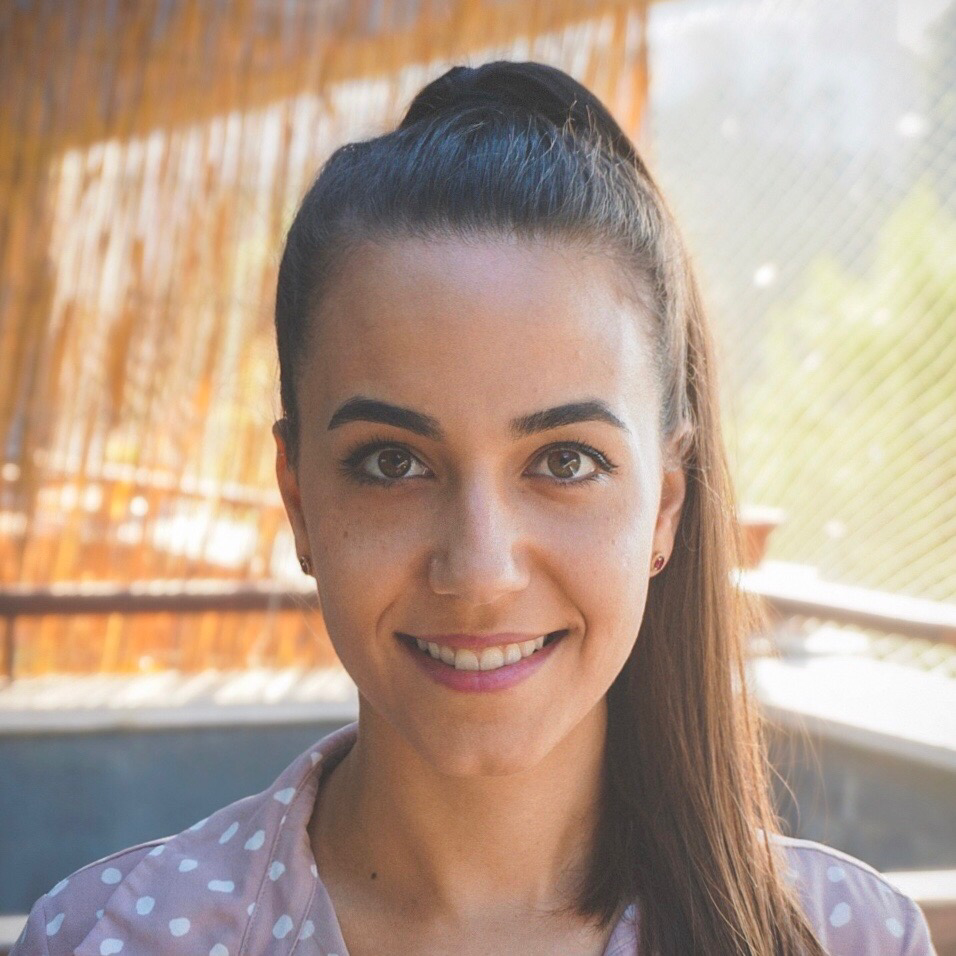
Roni Goldshmid
California Institute of TechnologyRoni is a postdoctoral research fellow working at the Dabiri Lab at Caltech. Motivated to unravel intricacies of fluid-structure interactions, she is studying visual data of vegetal kinematics resulting from wind loadings. The goal is to develop a wind speed prediction model from video footage of trees swaying in the wind. Before coming to Caltech, she completed her graduate studies at the Technion- Israel Institute of Technology where she focused on thermally driven atmospheric scale flows. More specifically, she focused on flows developing on mountains from diurnal solar heating.
Project: With wildfires more prevalent in the recent decades, firefighters could leverage aerial wind maps for fire mitigation purposes. This visual anemometry project leverages the dataset Roni collected in the last year. This dataset consists of both field and laboratory records that simultaneously sensed the wind with ultrasonic anemometers (on the ground) and the vegetal sway kinematics using an overhead camera. The field visual data was collected from a drone perspective, while the laboratory data was collected in a controlled wind tunnel using a stationary overhead camera.
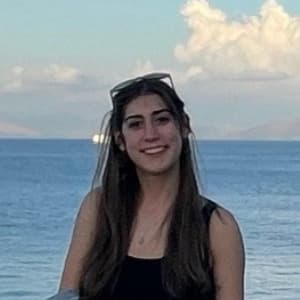
Natalie Imirzian (@nimirzy)
Imperial College LondonNatalie is a postdoctoral researcher at Imperial College London, where she studies the behavioral ecology of leaf-cutter ants. Ants form societies that rival our own, and her research focuses on how they have coordinated complex behaviors to become dominant members of our planet. She holds a B.S. in Biology and Evolutionary Anthropology from the University of Michigan and a Ph.D. in Entomology from Pennsylvania State University. During her doctoral work studying the behavior of fungal-infected ‘zombie ants’, she developed an interest in computational methods for studying behavior. Broadly, she is passionate about preserving biodiversity and making science accessible for all.
Project: Leaf-cutter ants form some of the largest and most complex social insect colonies, as millions of individuals work together to maintain a symbiotic fungus grown for food. Workers vary massively in size within a colony, but studying size variation proves tedious without automated methods. I aim to develop a scale-independent size detection tool that allows recognition of different sized workers in the field, as well as detects the leaf fragments they are carrying. This will improve our ability to study a major pest of the Neotropics, as well as improve monitoring of their consumption of different plants.
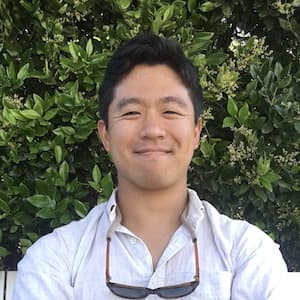
Brian Lee
University of California, Santa BarbaraI am a third year PhD student here at UCSB's Bren School of Environmental Sciences. My research involves studying interactions between human and natural systems through a suite of remote sensors. I am particularly interested in areas of data fusion and applying causal inference methods to better understand how we are affecting the landscape and vice versa. I hope to incorporate machine learning into my projects broadly. When I'm not working, you can find me surfing, fly-fishing, or on a long bike ride.
Project: My Summer CV project involves tracking bats in California's Central Valley using NEXRAD Doppler Weather Radar. My ultimate goal is seeing where these bats are spending time over the course of various time scales, from a single evening to decades, and exploring the relationship between where bats forage for pests and agricultural pesticide use.
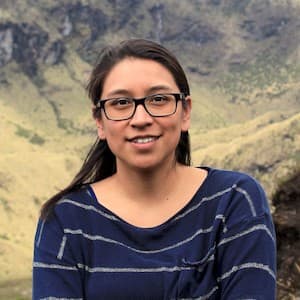
Francesca Ponce (@francesca_ponce)
California Institute of TechnologyI am Francesca, I am a grad student in biology at Caltech studying neurobiology in fruit flies. I study flight control mechanisms flies use to maintain stable flight. Previously, I worked on a series of release-recapture experiments in the Mojave Desert studying how flies disperse in an open area. I grew up in Quito, Ecuador, then traveled to Florida for college at the University of Florida.
Project: Long-distance dispersal is difficult to study in small insects, but with advances in remote sensor technologies, it is now possible to detect insects flying close to the ground. We performed release-and-recapture experiments using Drosophila and collected data to census the directional distribution of the flies as they disperse from a release site using sky-facing cameras. I seek to apply computer vision methods to these datasets to count the number of flies in each frame and track their trajectories as they move through the camera frame. This will inform existing models of how flies perform long-distance navigation, specially how they choose their initial headings while dispersing in an open landscape.
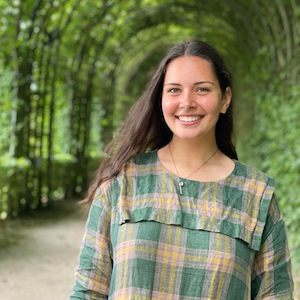
Alixandra Prybyla (@EdiBeeBrigade)
University of EdinburghAlixandra is a PhD student and Darwin Trust Scholar at the University of Edinburgh. As an undergraduate student at Columbia University, Alix developed a passion for non-lethal and non-invasive survey sampling methods, thus bringing her to her current work in acoustic monitoring. Her doctoral work focuses on how the flight sounds of the humble bumblebee can be used as a way to assess population dynamics–an especially pressing line of inquiry given that bumblebees are facing worldwide declines, and are important bioindicators. Alix is a firm believer that interdisciplinary collaboration can change the landscape of conservation ecology for the better, and she is excited to be an explorer of that horizon. She enjoys fire spinning and riding ponies across the Scottish moorlands in her spare time.
Project: Bumblebees face global population declines. As important pollinators, ongoing monitoring of population size, geographic distribution, and demography (i.e. age, sex, size) is essential for risk assessment and conservation. Because insect surveys are labor-intensive, automated and remote survey techniques--like acoustic monitoring--are of increasing interest. Bumblebees are excellent candidates for remote acoustic monitoring due to their distinct flight, foraging, and behavioral sounds. Therefore, the goal of this summer research is to train an AI-based verification system that can identify species-diagnostic signatures in a continuous stream of real-time sounds. Such technology would be a boon to people in the agricultural sectors, conservationists, and hobbyists alike.
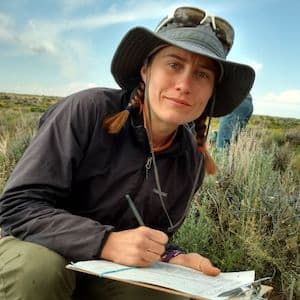
Rachel Renne
Yale School of the EnvironmentRachel grew up in rural southwest Florida, in the heart of orange and cattle-country. After graduating from college in 2008, she began a three-mile-per-hour tour—on foot—of the subtle and dramatic shifts of vegetation across the American landscape, hiking over 10,500 miles on National Scenic Trails. Rachel is a doctoral student at the Yale School of the Environment. She is fascinated by how soils translate climate into vegetation in drylands, and her favorite drylands are big sagebrush ecosystems. She is excited by the potential for combining traditional field data with artificial intelligence to answer fundamental and applied questions about plant community ecology.
Project: In rangelands, gradients of grazing intensity and disturbance form around water sources, with the greatest (and most visible) impacts close to the source, termed the “piosphere”. Ranching practices draw upon a suite of attractants (including artificial water sources) to achieve more even use of forage across the landscape. Yet, data sources for these attractants are incomplete and inaccurate. My project will use computer vision to detect piospheres in Wyoming using aerial imagery. This project will provide critical data to identify water- and livestock-remote areas, with important applications for land management, conservation, and sample design for research (like mine) that addresses fundamental questions about the interacting roles of environmental factors and disturbance in determining dryland plant community structure and function.
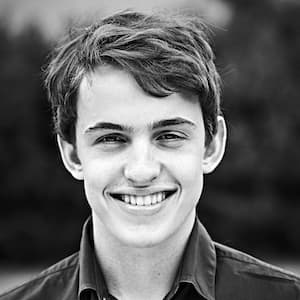
Felix Rustemeyer (@FelixRustemeyer)
Maastricht University / Stockholm Environment InstituteI am Felix Rustemeyer, a dutch-german graduate student from Maastricht University in the Netherlands. After conducting a bachelors in Artificial Intelligence at the University of Amsterdam, I am now finishing my masters in Data Science for Decision Making. Inspired by a minor in Geomatics during an exchange in Sweden, I have grown a passion for employing the power of deep learning and computer vision for raising awareness and tackling the issues of our time. In an internship role at the Stockholm Environment Institute, I have conducted this last semester by using deep learning for identifying the impact of permafrost thaw. I am honored to be part of the inaugural cohort of the CV4Ecology summer school and am extremely excited for August!
Project: Thawing permafrost is a huge problem as permafrost soils are heavily carbon rich and is therefore causing large amounts of GHG emissions, while being absent from many international dialogues and strategies aimed at tackling climate change. The Stockholm Environment Institute has established a cross-sector collaboration to fill in this knowledge gap. The goal of this project is to identify landscape indications of thawing permafrost in the arctic using computer vision and satellite imagery. By identifying and monitoring these landscape indications in the form of thaw slumps, the extent, increase and the impact on climate caused by thawing permafrost in the arctic can be estimated.
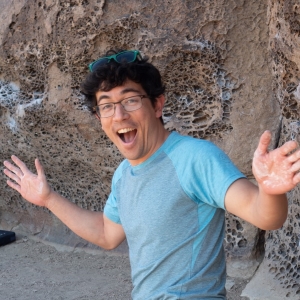
Taiki Sakai
Environmental Assessment Services / SWFSC Acoustic Ecology LabTaiki is a contractor at NOAA’s Southwest Fisheries Science Center working in the Acoustic Ecology Lab. There he works with researchers who use passive acoustic monitoring to study marine mammals off the coast of California. His main focus is building free, open-source software tools that make it easier to analyze passive acoustic data. His favorite animals are beaked whales and frogfish because they are adorable weirdos. When he’s not behind a computer, you can find him hiking, climbing rocks, or playing with his dog, Noodle.
Project: Beaked whales are deep diving marine mammals that are difficult to study using traditional visual methods because of how little time they spend at the surface. During their long foraging dives they make echolocation clicks which can be uniquely identified to different beaked whale species, which makes them an ideal candidate to study using passive acoustics. In this project we will create images of echolocation clicks using the Wigner-Ville transform and attempt to classify them to species.
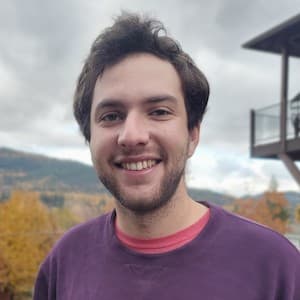
Ethan Shafron
University of Montana Spatial Analysis LabI am an analyst and field coordinator for the University of Montana Spatial Analysis Lab in. After earning my Bachelors degree in Environmental Studies from University of Vermont in 2019, I worked on geospatial scientific computing and imaging spectroscopy at Arizona State University. My work there involved mapping coral reefs, foliar functional trait mapping, and survey design for marine biodiversity monitoring. I then spent a winter season working with Glacier National Park's Citizen Science program helping to create wildlife surveys and analytical products for improving park management and visitor experience. I am interested in spatial ecology, land system science, algorithm development, and machine learning.
Project: We are working to characterize woodland draws and ravines across a huge area of Eastern Montana and the Dakotas in conjunction with the Bureau of Land Management. These systems are critical for wildlife and grazing, and are vital to the health of the region and function of its agriculture industry. These systems are particularly difficult to monitor due to their narrow, dendritic shape, so we will be using computer vision methods and remote sensing to develop a scalable monitoring protocol.
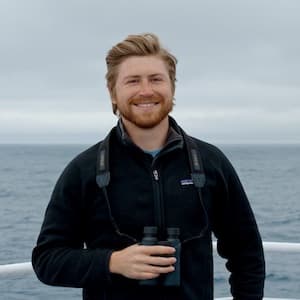
Casey Youngflesh (@CaseyYoungflesh)
Michigan State University (as of Aug 1, 2022)I am a quantitative ecologist and Presidential Postdoc Fellow in EEB at Michigan State University (as of August 2022). My research uses a range of quantitative approaches that synthesize large-scale data streams to address both basic and applied questions in population and community ecology. I seek to understand how and why ecological systems are responding to rapid climatic change and what this might tell us regarding how best to conserve these systems. I have a particular interest in the timing of seasonal events (phenology), population dynamics, and biodiversity.
Project: The Pacific walrus is an important benthic predator in Arctic marine ecosystems and a species of substantial cultural and economic importance to many Indigenous Arctic coastal communities. As a result of increasing pressures associated with global change, the future of this species is highly uncertain. I aim to develop a deep learning-based classifier to identify walrus in satellite imagery. This will serve as the basis for large-scale, automated efforts to monitor the distribution and abundance of this species and will provide stakeholders with information needed to make timely conservation management decisions.