Students 2023
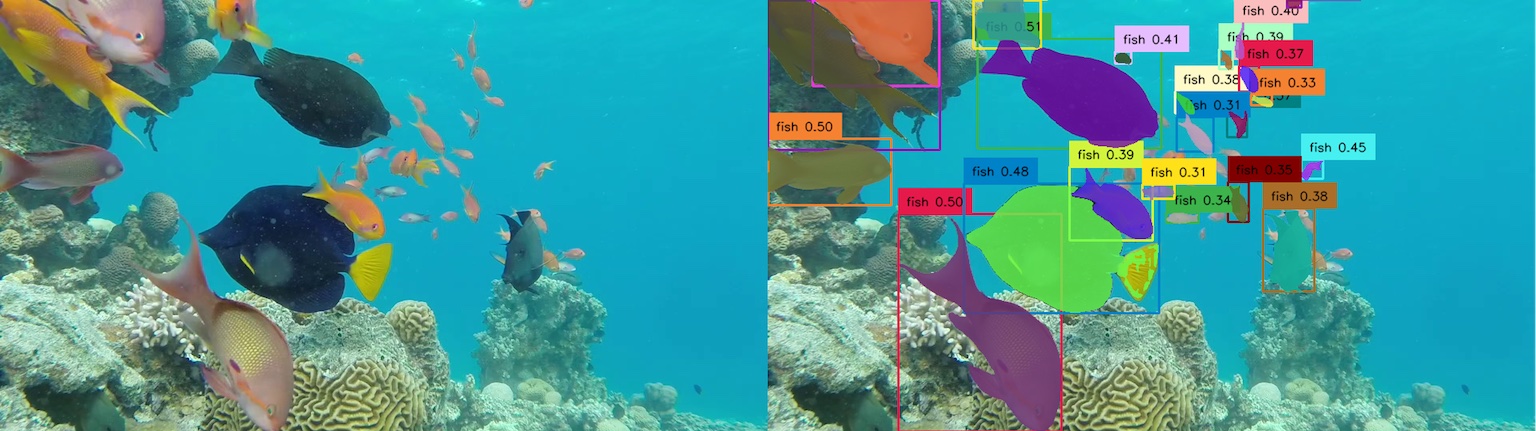
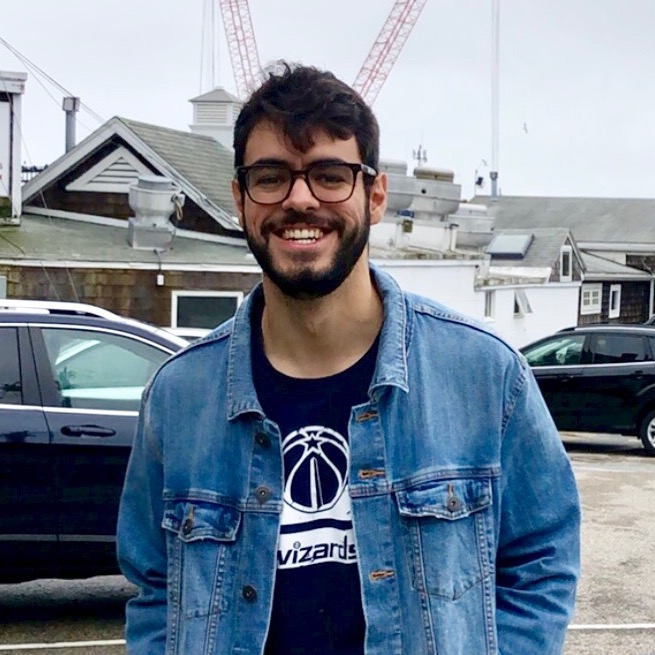
Vinicius Amaral
University of California, Santa CruzI'm a PhD student in the Ocean Sciences department at the University of California, Santa Cruz. My doctoral research focuses on using computational methods to estimate the rates at which carbon is cycled in the ocean, but I'm broadly interested in applying data-driven methods to solve problems in the natural environment. Before venturing into oceanography, I studied civil and environmental engineering at Princeton University.
Project: The ocean influences the concentration of carbon dioxide (CO2) in the atmosphere, partly due to a network of biologically mediated processes known as the biological carbon pump. Photosynthetic algae fix CO2 into biomass in the surface ocean. As algal cells collide and coalesce into aggregates or are repackaged into fecal pellets by zooplankton, they become part of the marine snow that settles through the water column. I'm working on a computer vision-based method to identify marine snow particles and estimate the carbon fluxes that they contribute in order to better constrain the ocean’s role in the global carbon cycle.
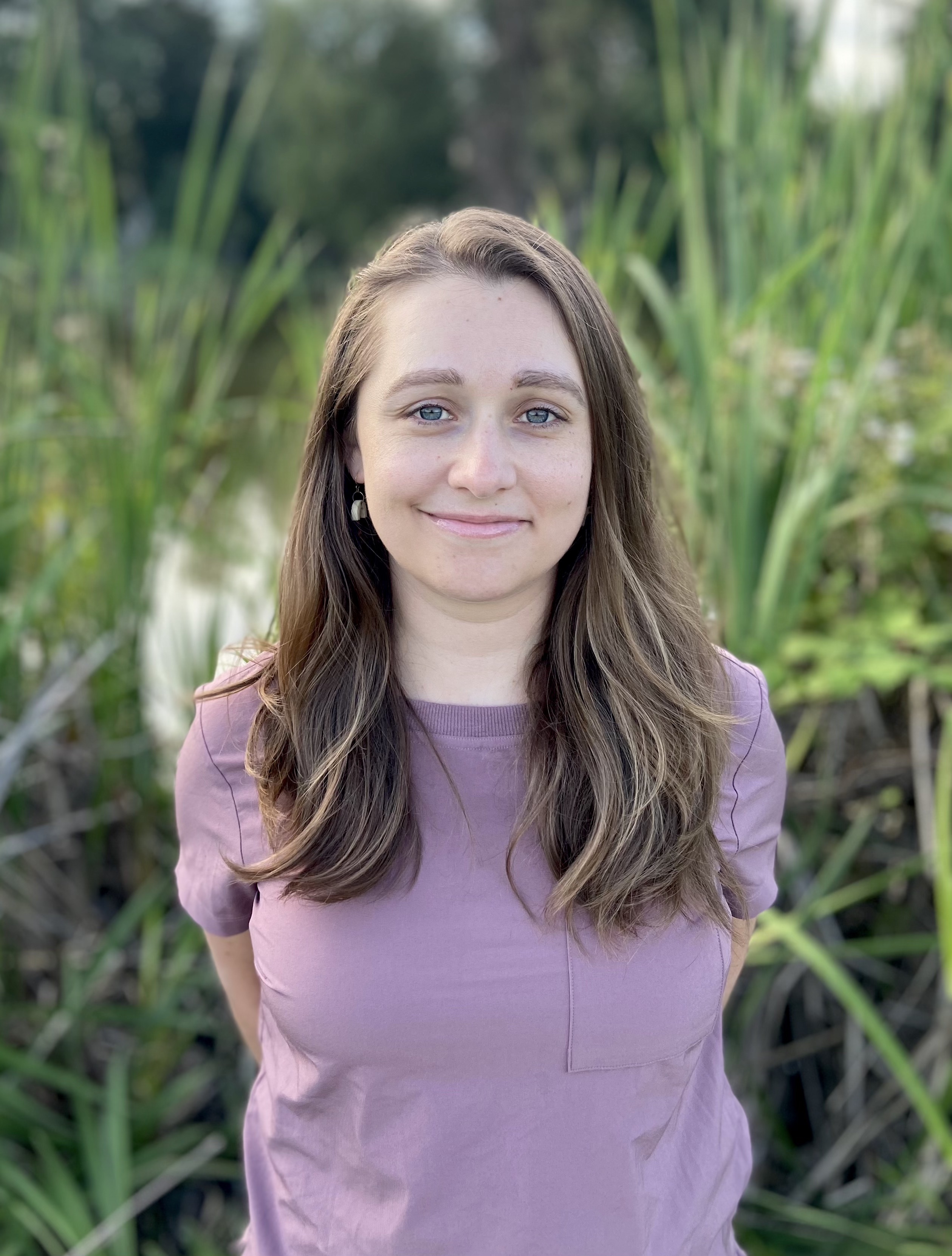
Cara Appel
Oregon State University and the US Forest ServiceCara is a PhD student in Wildlife Science at Oregon State University and an affiliate with the US Forest Service. Her dissertation is focused on applications of machine learning to wildlife research and conservation, including passive acoustic monitoring for northern spotted owls and remote camera trap projects in Oregon and Malawi. Cara has a B.A. in environmental studies from the University of Washington and an M.S. in wildlife from Cal Poly Humboldt. In her free time, Cara likes to read and explore nature.
Project: The Pacific Northwest of North America is one of the largest timber-producing regions in the world, and being able to effectively monitor forest biodiversity in the region is essential for management and conservation. The recent establishment of a large-scale bioacoustics monitoring program in the region, with thousands of sites visited every year, has laid the groundwork for complementary projects, including using remote cameras to survey forest wildlife communities. The goal of this project is to develop a computer vision model to identify wildlife species from camera trap images, using training data collected over several years from hundreds of sites in western Oregon.
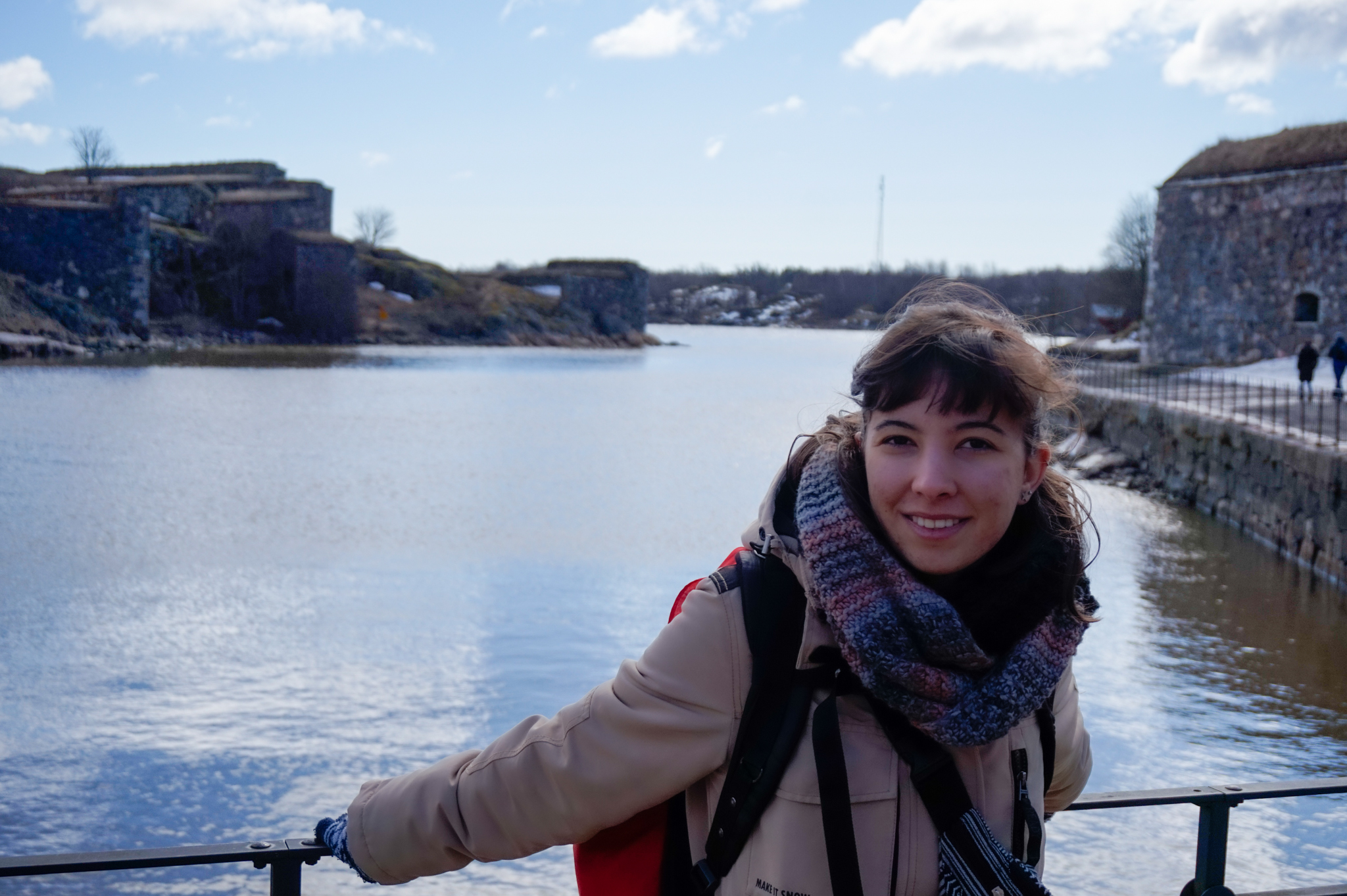
Alba Márquez-Rodríguez
University of HuelvaMy name is Alba Márquez-Rodríguez, and I am a Computer Engineer from the University of Huelva. Combining my passion for computer science with my deep environmental awareness, I have discovered a strong interest in the intersection of technology and ecology. With a background in computer science, I am eager to contribute my skills and knowledge to the field of Artificial Intelligence for conservation purposes. Through my involvement in environmental organizations and my current project at Doñana National Park, I am driven to make a positive impact on biodiversity conservation and promote the collection of non-invasive data for accurate monitoring.
Project: I am currently working on an ongoing project titled "Automated census and biodiversity monitoring system using deep learning techniques." This project aims to develop a cutting-edge protocol and workflow for wildlife monitoring, leveraging camera trapping, citizen science, and Deep Learning. My role within the project involves creating a Neural Network for species classification using camera-trap images from Doñana National Park. By developing advanced techniques and optimizing code efficiency, our goal is to obtain unbiased estimates of species and community dynamics, enabling cost-effective and prompt responses to ecological changes. I am excited about the opportunity to further my knowledge and skills through the CV4Ecology Summer Workshop and contribute to the advancement of AI in ecology.
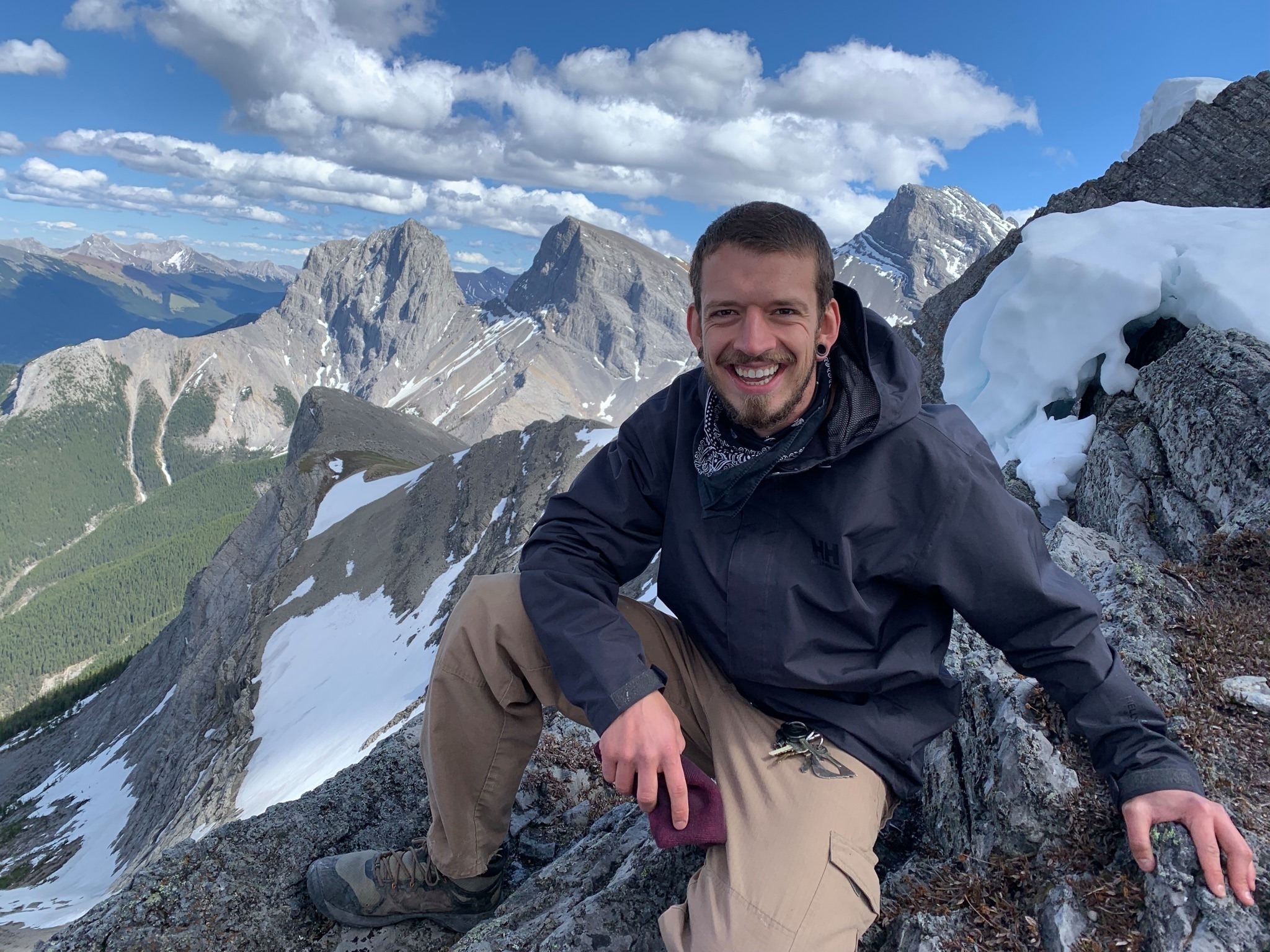
Michael Procko (@xprockox)
Research scientistI am a contractor working with the Tulalip Tribes of Washington State, was previously a Research Scientist in the Prugh Lab at the University of Washington, completed a MSc in the Wildlife Coexistence Lab at the University of British Columbia, and obtained a BA in Ecology and Evolutionary Biology from the University of Colorado. My interests lie at the intersection of wildlife conservation and conflict mitigation, where I use novel analytical approaches to bridge the gaps between the biological sciences, land use policy planning, and wildlife management. When I’m out of office, I am likely prioritizing my mental health (planning backpacking trips and tattoos) and advocating for others to do the same (however they see fit—tattoos not necessary).
Project: I am hopeful to work towards real-time human-wildlife conflict mitigation by developing predator detection models that can run in-house on satellite- or cellular-linked camera traps, processing images in real time and sending alerts to land and wildlife managers when predators enter areas of high potential conflict (e.g., recreation hotspots, agricultural landscapes).
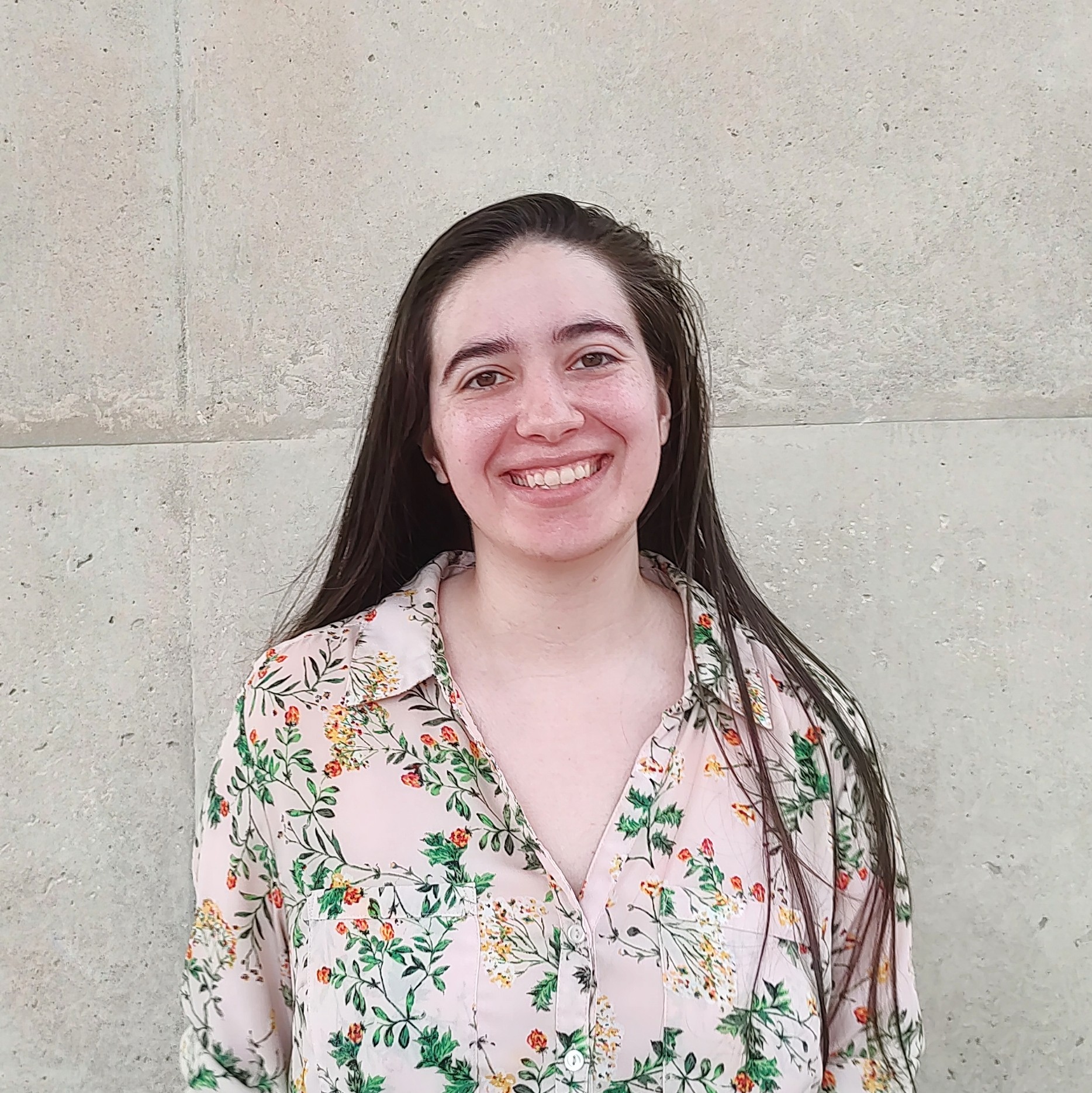
Niah Holtz
University of Massachusetts, AmherstI am a Ph.D. student at UMASS Amherst in the Organismic and Evolutionary Biology program. I study phenotypic and functional shifts resulting from rapid radiations in African cichlids and repeated evolution in Astyanax mexicanus cavefish. My focus is on curating and analyzing high-throughput behavioral and genetic data. Machine learning is a robust tool for processing high-throughput visual data, and I hope to utilize it throughout my career and collaborations. I dedicate myself to increasingly integrative and accessible science and seek to contribute to ecological and organismic health. In my personal time, I enjoy a good resource management game or mountainside picnics. If you see me around, I am always willing to find a good snack and talk about science.
Project: As the importance of biodiversity is increasingly understood, so are the mechanisms by which it is created and maintained. Interactions between selective pressures across time and at every level of cellular organizations contribute to organismic traits. Our species of interest, Lake Malawi cichlids, display an impressive diversity in functional morphology within a relatively recent rapid radiation. We ask whether the genetic architectures surrounding the timing of kinetic behaviors in cichlid species contributed to—or supported—the levels of functional biodiversity seen in cichlid populations through temporal niche partitioning. If so, does the timing of kinetic behavior change given environmental cues like light, sediment, novel objects, or conspecific organisms?
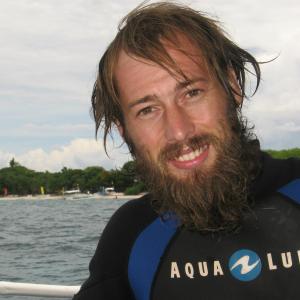
Christopher Roberts
University of New South Wales, SydneyI am a PhD candidate at UNSW Sydney, Australia, where I have integrated my passion for diving and underwater photography with my research by looking at the potential use of recreational dive photographs to monitor marine species and their habitats. As part of my research I created an iNaturalist project, In Bygone Dives to collect old underwater photographs from the SCUBA diving community as a baseline for marine ecosystems from which to track change.
Project: My project is looking at the potential value of incidental data captured in the background of photographs as a source of data to monitor marine habitat distribution. Specifically, we hope to use photographs shared to iNaturalist, but rather than assessing the subject of the observations (i.e., fish) we plan to use computer vision to classify the reef captured incidentally in the background as an alternative technique for broad scale marine habitat mapping and monitoring.
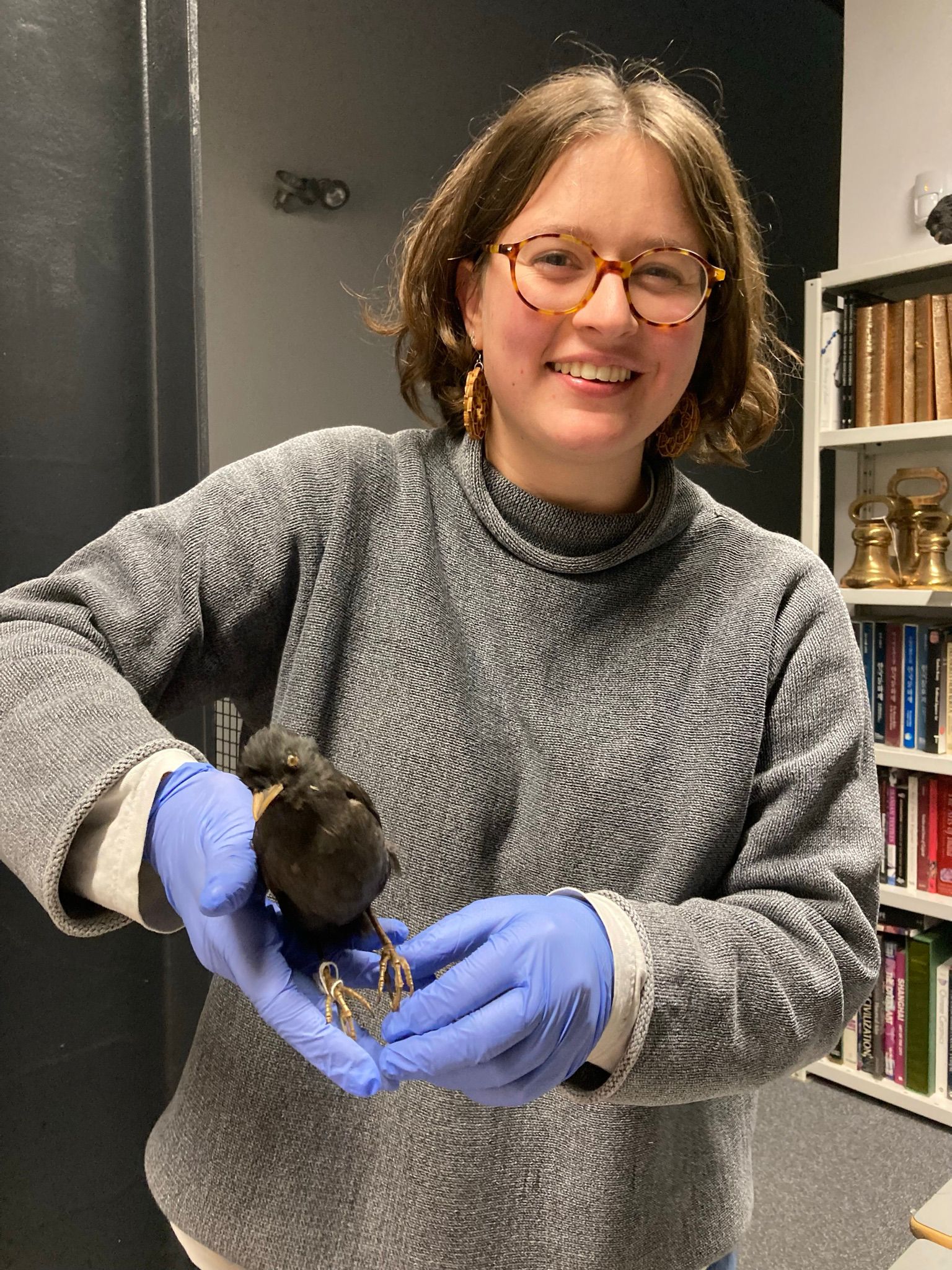
Sicily Fiennes (@sicilyfiennes)
University of LeedsI'm Sicily (they/them), a second-year PhD student at the University of Leeds in the Leverhulme Extinction Studies Program. My doctoral research focuses on the Asian Songbird Crisis as a phenomenon, particularly in the global songbird trade hotspot, Indonesia. I am a conservation biologist by training but have now shifted to a more interdisciplinary research focus, working across the various fields of conservation social science, political ecology, computer science and design. Outside of PhD life, I love playing football (Arsenal till I die!), bouldering and making silly little things at home like limoncello, candles and soap!
Project: In Southeast Asia, hundreds of species of songbirds are sought after for singing competitions, breeding, prayer release, and as pets. However, the sheer diversity of species involved poses a fundamental challenge for identification and monitoring. The main project I will explore at the CV4Ecology Summer School is a machine learning-driven image classifier for species sold in bird marketplaces in East Asia, targeted at law enforcement through a mobile phone application. I am also interested to see if one or few-shot learning networks can be used for rare, data-poor species.
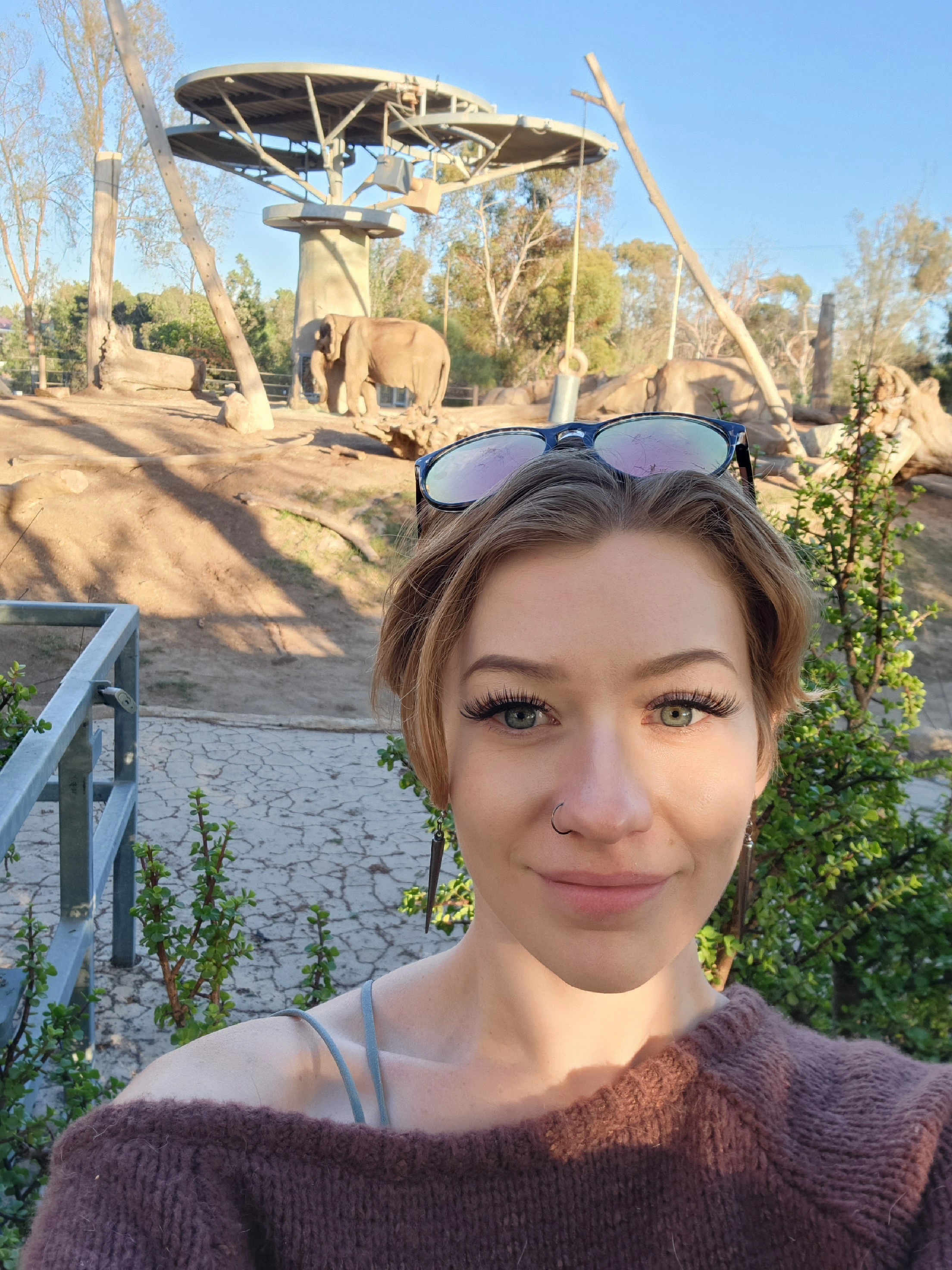
Kyra Swanson
San Diego Zoo Wildlife AllianceI am a Research Coordinator II at the San Diego Zoo Wildlife Alliance. After completing my PhD in Behavioral Neuroscience at American University, I did postdoctoral research on elephant foraging behavior and gait analysis using CV tools at the Smithsonian National Zoo. This led me to my current position at SDZWA, where I apply a number of machine learning techniques to image processing and data analysis. I maintain AniML, a software package in Python and R for model training and species classification of camera trap images.
Project: Species classification of camera trap images alone cannot tell us how many of that species are in a given area. I will be continuing the development of Uniqorn, our unsupervised clustering algorithm for re-identification of patterned species such as jaguars, tigers, and giraffes. This algorithm improves on current methods that require a human-labeled database for reference.
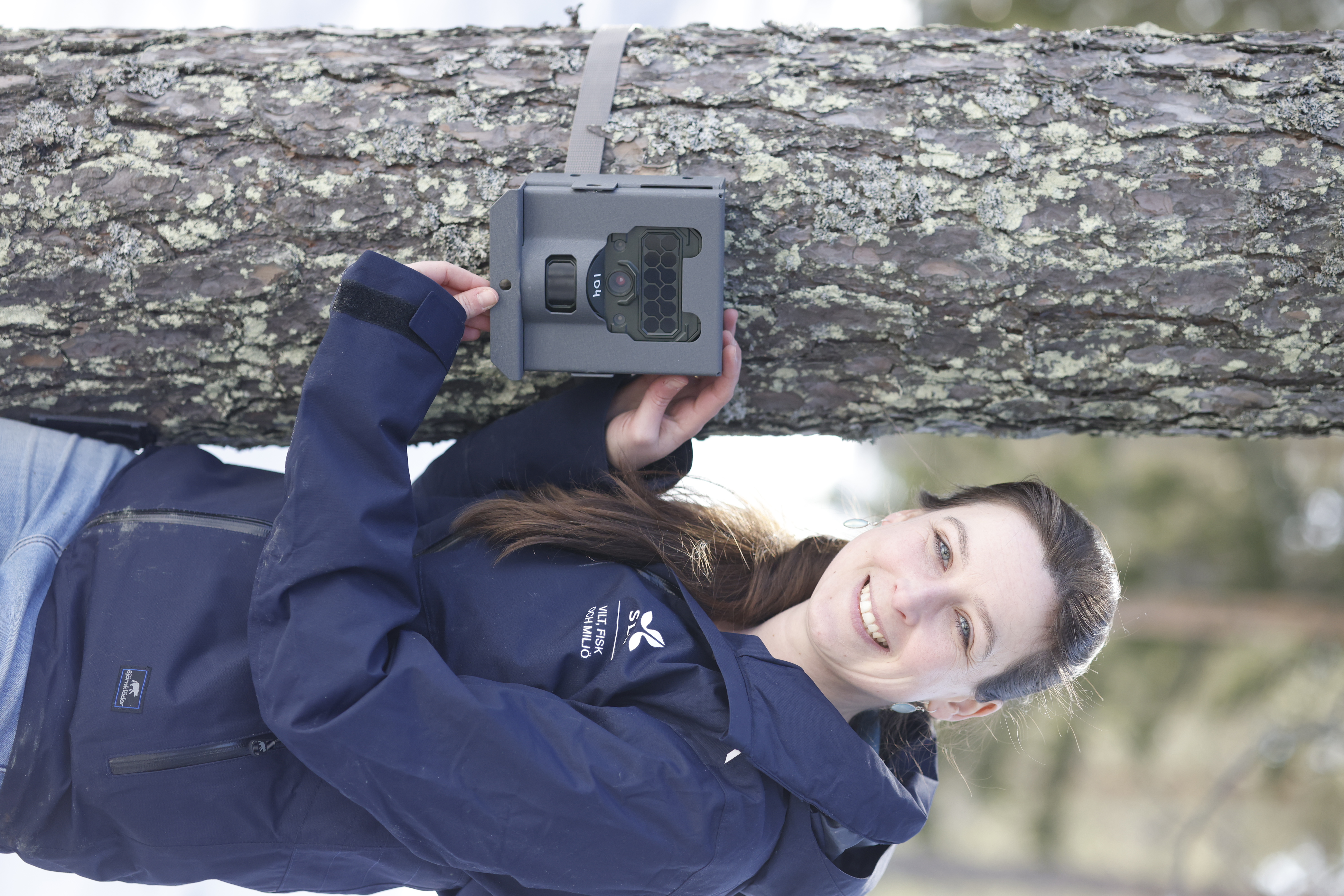
Magali Frauendorf (@M_Frauendorf)
Swedish University of Agricultural SciencesI am a post-doctoral researcher at the Swedish University of Agricultural Sciences (SLU) (at the Department of Wildlife, Fish and Environmental Studies) in Umeå, Sweden. In my current research, I apply technologies around camera trapping and AI as well as citizen science to study ecological questions related to wildlife conservation and management. Before starting my post-doc at SLU, I conducted my PhD at the Netherlands Institute for Ecology. I investigated the reproductive ecology of inland breeding oystercatchers by studying the main drivers of the reduced reproductive success in the breeding area itself as well as potential carry-over effects of winter condition on the performance in the breeding season on a large national scale. In general, I have an academic background in reproduction and quantitative ecology with a strong interest in movement, population and conservation ecology. My research is characterized by combining citizen science, advanced statistical methods and different monitoring techniques/technology to study large-scale ecological relationships.
Project:I am involved in the Viltbild program, where SLU collaborates with the Swedish Association for Hunting and Wildlife Management. With Viltbild, we develop a platform to collect and organise camera-trapping images taken by hunters all over Sweden. On a long-term, this can serve as a digital monitoring tool to monitor Swedish game species with the help of hunters. During the CV4E summer workshop I will work on building algorithms that can distinguish sex and age (juveniles, adults) on camera trap images from five ungulate species (occurring in Sweden). An automatic identification of sex and age can help us to estimate reproductive performance and in turn result in a quicker feedback loop between monitoring and management, leading to management that is more appropriate.
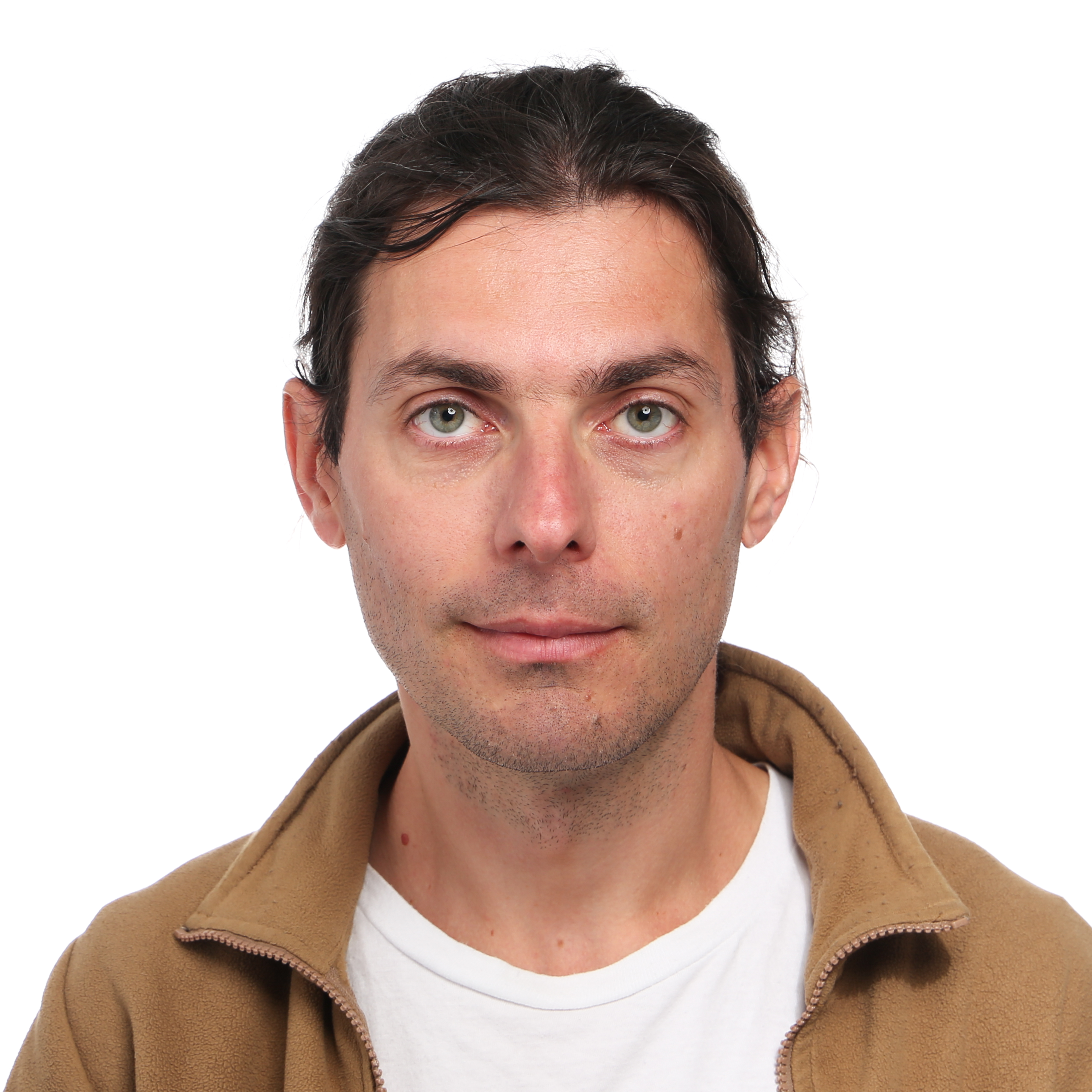
Valentin Ștefan
Helmholtz Centre for Environmental Research (UFZ) & German Center for Integrative Biodiversity Research (iDiv) Halle-Jena-Leipzig.My professional journey has led me from hands-on forestry work to the intricate world of research, currently delving into the exciting realm of data analysis and computer vision in the field of ecology. I'm driven by the thrill of solving data puzzles and answering research questions using state-of-the-art open source tools and programming. My prior experience involves working with a dedicated team of pollination ecologists, aiding them with R programming for their research. The current focus of my research work is an AI project that aims to automate data collection on plant-pollinator interactions.
Project: Pollination is critical to many ecosystem services and to human nutrition. Currently, pollination ecologists spend countless hours collecting, killing and then identifying pollinators in the lab in order to acquire enough data to understand plant-pollinator interactions and their change across environmental gradients and anthropogenic disturbances. Such expensive data collection approaches could be automated by using novel tools from technology and computer vision. The key question driving my work is: "How can we optimally harness the power of AI to automatically collect and analyze data and images of how plants and pollinators interact?"
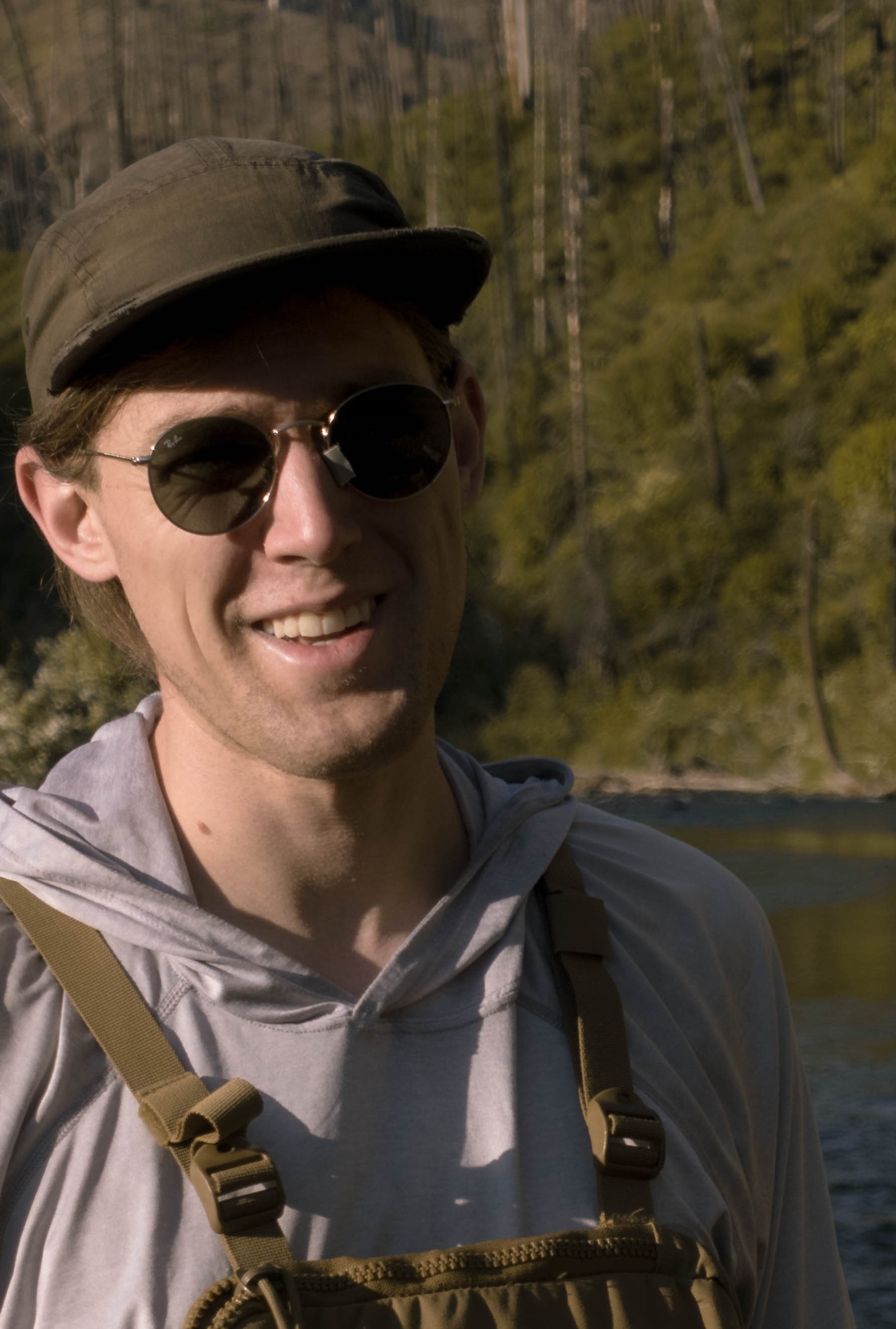
Michael Getz
Oregon State UniversityI'm a master's student in the Horticulture department at OSU with a BA in International Area Studies from New College of Florida and a BS from OSU in Botany. In between degrees I've worked as an English teacher, furniture maker, copywriter, and backcountry botanist. My interests in design, field ecology, and data science intersect at the development of techniques for automated insect monitoring. Off the job I'll be woodworking, fly fishing, looking at plants, or keeping our old trucks running.
Project: Pollinator health is critical to the maintenance of agricultural and ecological systems, though our current methods for surveying pollinators are resource-intensive and data-poor. Developing a method for continuous, long-term monitoring of pollinator activity could improve understanding of species distributions, pollinator guilds, and how population dynamics are affected by stressors such as human impact and climate change. I'm working to use Deep Learning techniques to effectively process images from automated insect camera traps.
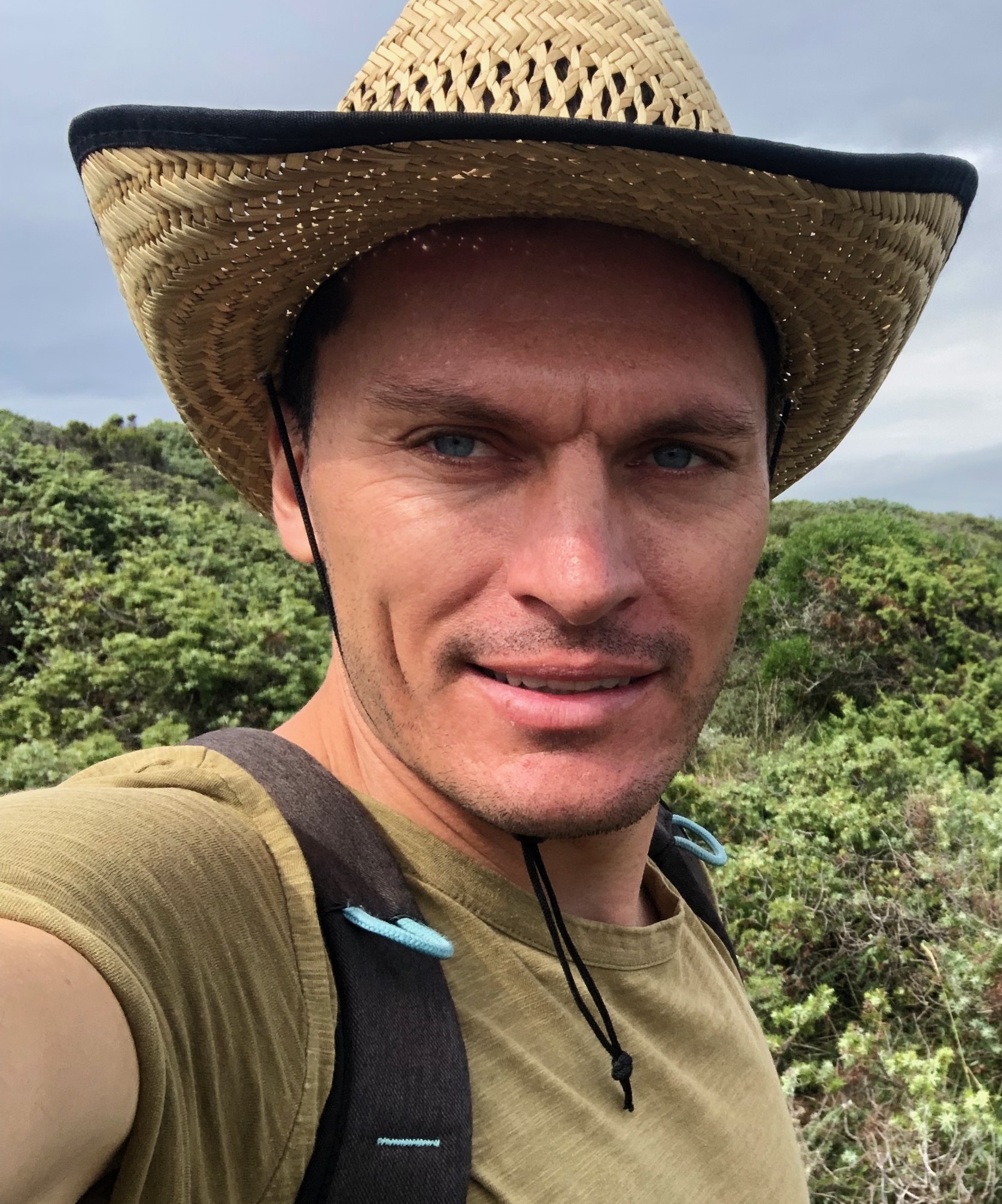
Martin Marzidovsek
Jozef Stefan International Postgraduate School (IPS)I am a research assistant at the Department of Knowledge Technologies at the Jožef Stefan Institute in Slovenia where I have applied machine learning (ML) in various ecological research. Currently, I am completing my Ph.D in ML at the Jozef Stefan Postgraduate School on the topic Explainable Machine Learning on Heterogenous Data in Life Sciences. Before that I co-founded a venture capital backed tech company that was selected for the leAD business accelerator in Berlin an I hold a Master’s degree in International Affairs from the University of Geneva.
Project: Diversity of phytoplankton is essential for Marine ecosystems' processes (production of organic matter, pelagic food webs, carbon pump) and fate of these processes depends critically on phytoplankton community structure. It is thus important to investigate how changing physical and chemical sea conditions due to climate change and other anthropogenic pressures impact the phytoplankton physiology and community structure and thus disrupt the marine ecosystem and its functions. The aim is to develop a computer vision system that can automatically identify and count phytoplankton species of interest, estimate cell size and calculate the biovolume of the specimen.
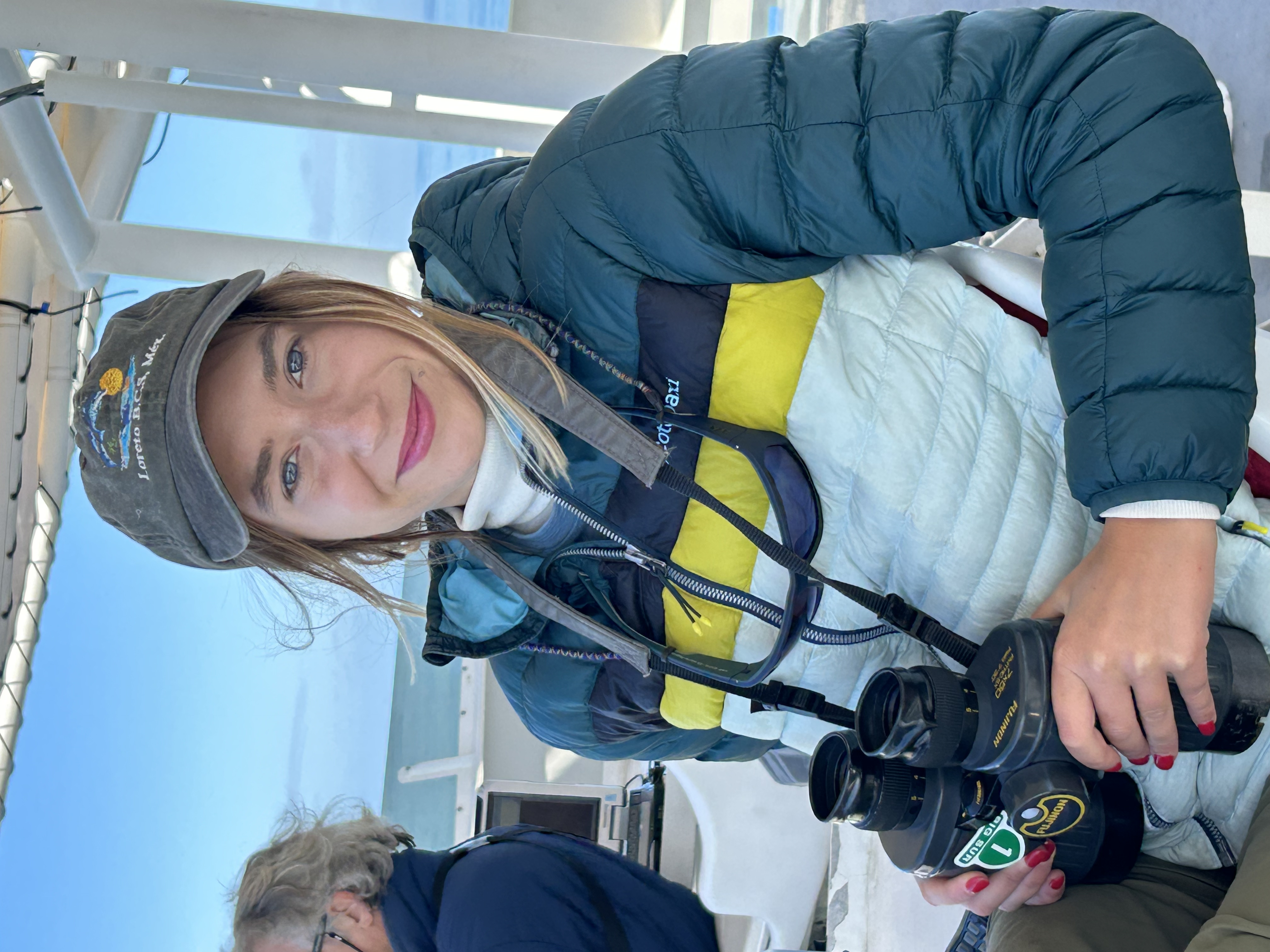
Michaela Alksne
Scripps Institution of OceanographyMichaela (she/her/hers) is a PhD student and NDSEG Fellow at Scripps Institution of Oceanography. Her dissertation research focuses on applying novel machine learning tools to improve underwater acoustics data processing. Trained as a marine mammal biologist, Michaela’s core research goals are to understand cetacean (whales, dolphins, and porpoises) behavior, distribution, and foraging ecology, using acoustics. To do just this, Michaela is excited about applying computer vision tools to detect and classify unique cetacean signal types in spectrograms. When she’s not listening to whale songs or wrestling matlab and python, you can usually find her surfing or swimming nearby.
Project: Blue whales come to Southern California in the summer to forage on dense patches of microscopic krill. While in the region, they produce low-frequency foraging, social, and reproductive calls. Using bottom-moored acoustic sensors, we have a long-term record of their singing behavior at multiple sights, giving us insight into their seasonal, diel, and interannual behavioral patterns. However, given the large volumes of data we collect, detection of blue whale songs is like finding needles in a haystack. There is currently no reliable method to detect and classify highly variable blue whale social and foraging calls. For CV4Ecology, Michaela is bringing a training dataset of blue whale call spectrograms in an effort to develop a robust deep learning model that is capable of detecting and classifying complex, highly variable, northeastern pacific blue whale calls in passive acoustic data.
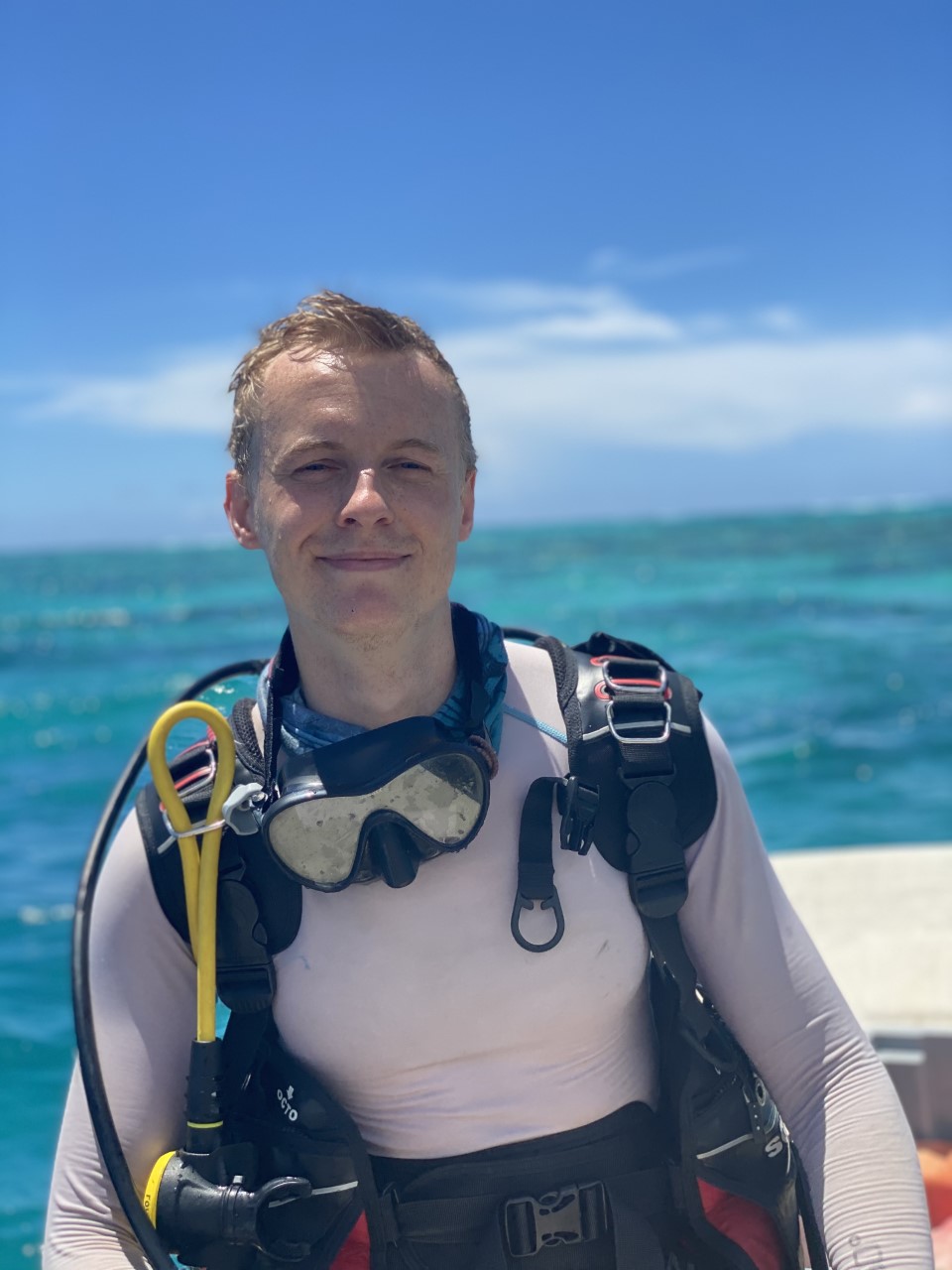
Ben Williams
University College London and Zoological Society of LondonHi I’m Ben, a PhD student at UCL and ZSL in the UK, supported by the Fisheries Society of the British Isles. My PhD is focused on leveraging machine-learning to monitor and support coral reef conservation. This is in close cooperation with my industry partner Mars, who operate the world’s largest coral reef restoration program (buildingcoral.com). I have a strong background in marine biology and have enjoyed the continued opportunity to boost my skillset in machine learning over the course of my PhD.
Project: The soundscape of coral reefs is a rich and diverse symphony of different noises. An increasing body of research has shown there is much we can learn about the state of these habitats by listening. Machine learning presents the cutting edge in similar domains, but is so far underutilized on reef soundscapes. My project aims to utilize a large and diverse dataset of reef soundscape recordings from across the tropics to develop a first prototype of a foundational model that can be used as a springboard for ML led analysis of reef acoustic data. This model should enable users to extract domain specific feature embeddings from reef audio, enabling the training of unsupervised clustering algorithms or shallow classifiers with most of the time and compute cost removed.